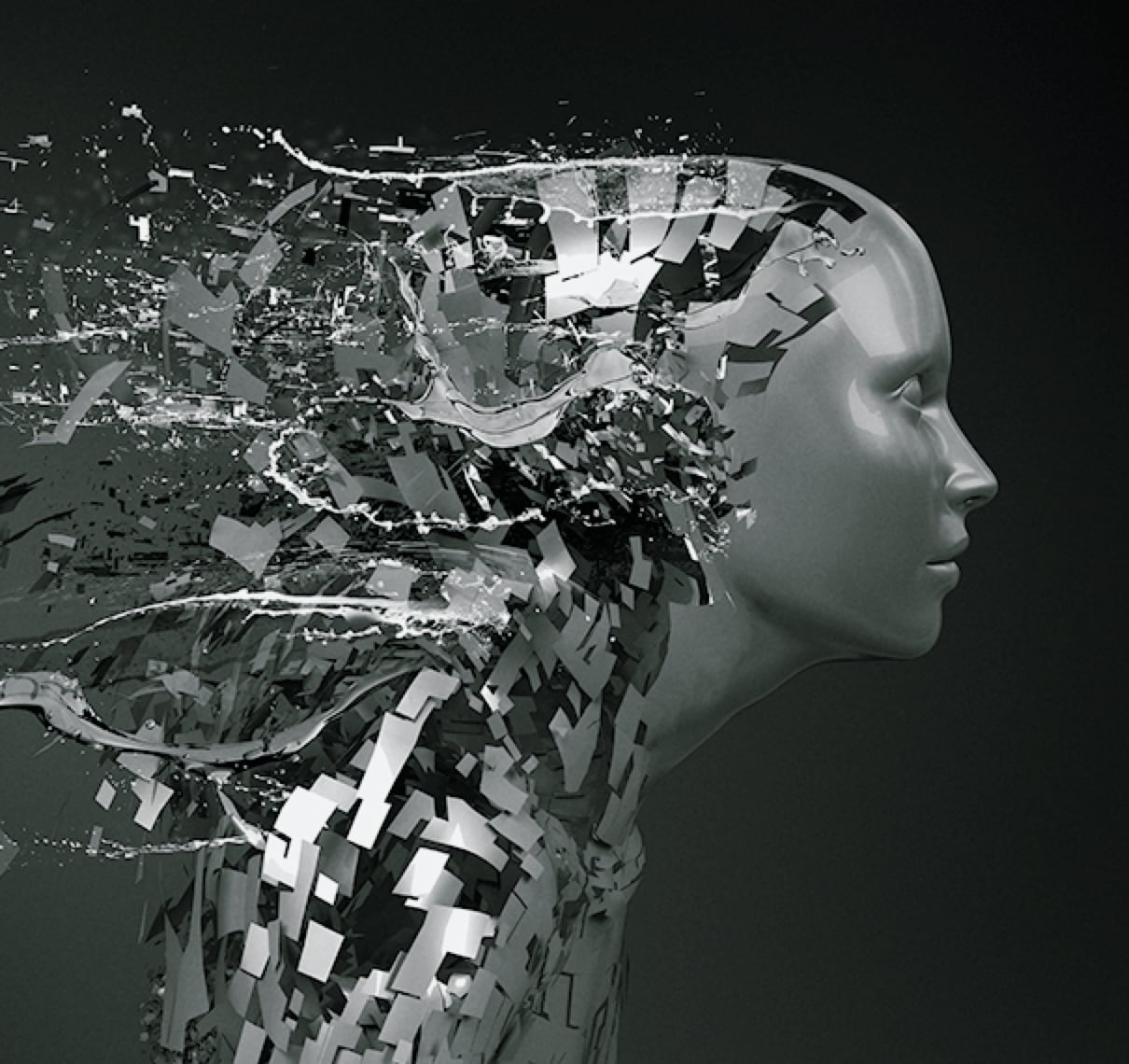
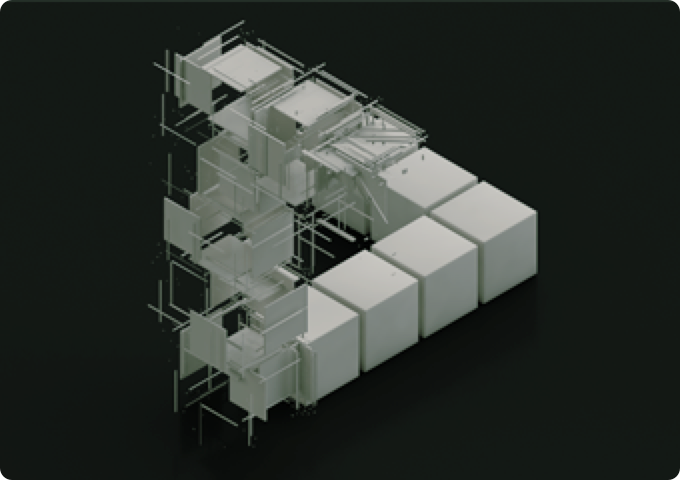
Summary
Today, AI is a disruptive technology in the digital era. But every coin has two side. While AI technologies in the cybersecurity domain provide significant benefits in increasing robustness, it can also be used maliciously by hackers to commit massive security breaches. This article aims to discuss how the misuse of AI is taking place, how ML and DL technologies aid hackers in planting sophisticated attacks, and how resilient systems should be built to evade such attacks.
AI has emerged as a disruptive technology in this digital era. The magnitude of this super technology can be understood by the tech tycoon Elon musk’s statement, “I fear the dominance of AI.” As technology and innovation are advancing rapidly, the threat environment is also increasing; more innovative, sophisticated cyberattacks are prevalent by hackers utilizing AI technologies like Machine Learning models and Deep Learning capabilities. As every coin has two sides, AI technologies in the cybersecurity domain provide significant benefits in helping organizations build more resilient secure systems and tools but can be used maliciously by hackers to commit security breaches which will have a devastating impact on various targeted organizations/countries across the world.
This article aims to discuss how the misuse of AI is taking place and also how ML and DL technologies aid hackers in planting sophisticated attacks, and how resilient systems should be built to evade such attacks. Furthermore, I will be writing about how these technologies help hackers/organizations build security tools to identify critical vulnerabilities which are otherwise hard to find using traditional tools and techniques.
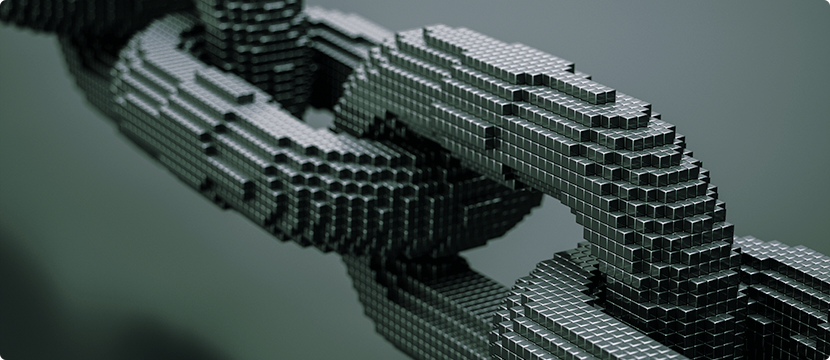
Misuse of AI
In today’s Information Age, the amount of data we produce on a daily basis is truly overwhelming. AI uses Machine Learning and Deep Learning algorithms to process large amounts of data, learns from the data, and ultimately solves worldly problems. The more it learns, the stronger it is!
AI has become an integral part of our day-to-day lives. Unquestionably, everyone has their reservations, whether AI-based systems are vulnerable to cyberattacks or not? Most of us employ Machine Learning technologies in our daily life when we use search engines like Google and Yahoo. Whether it is voice assistants like Alexa, Siri, or OKGoogle, or recommendation systems on Netflix, YouTube, or even e-commerce portals, the application of AI is in almost every field.
Let’s see how Google, Facebook, and Twitter use AI technology to serve the relevant feed to their users. Inevitably, it raises a lot of privacy concerns too. These platforms collect information (view history, search history, clicks, hovers etc.) to promote certain ads to influence them. This is also called mental malware used by tech giants to influence user decisions. The perfect example of misusing AI by tech giants is Cambridge Analyticaⅰ —Facebook data privacy scandal. The Facebook–Cambridge Analytica data scandal concerned obtaining the personal data of millions of Facebook users without their consent by British consulting firm Cambridge Analytica, predominantly to be used for political advertising.
Now let us understand the possible misuse of AI against automated speech recognition (ASR) systems. ASR is the technology that enables voice technologies like Amazon Alexa, Apple Siri, OkGoogle, and Microsoft Cortana to parse voice commands. A malicious intent user might tweak a music file, post on YouTube to contain a hidden voice command. This YouTube link can be sent as a phishing link for a targeted victim to click. When the song is played by the victim using voice technology, in general, humans won’t notice the hidden command while the song is being played; it would be clearly audible to Machine Learning algorithms which look for patterns in sound waves and can act. The possible damage could range from canceling your daily routines, deleting trained skills & history of voice commands on these voice assistant devices, and further exploiting weaknesses present in other smart technology integrations like a smart lock, smart home, etc.
Fooling Machine Learning Models
If we don’t build resilient and secure AI systems, it might have a catastrophic effect on organizations and humans who use these AI-based systems. Security researchers tricked a Tesla Model S into switching lanesⅱ in March 2019. All they had to do was place a few unobtrusive stickers on the road. This technique exploited a weakness in the Machine Learning algorithms that power Tesla’s Lane Detection technology in order to cause it to behave erratically.
In another instance, researchers demonstrated how an attacker could fool the image processor of a self-driving carⅲ into bypassing a stop sign or mistaking it for a speed limit sign. Just imagine the potential damage it can cause to human lives if things go out of control like this.
There are many such adversarial misuses of AI systems possible in the Intelligence financial fraud detection systems, smart healthcare systems, etc. The malicious actors in such cases can be insiders or external hackers.
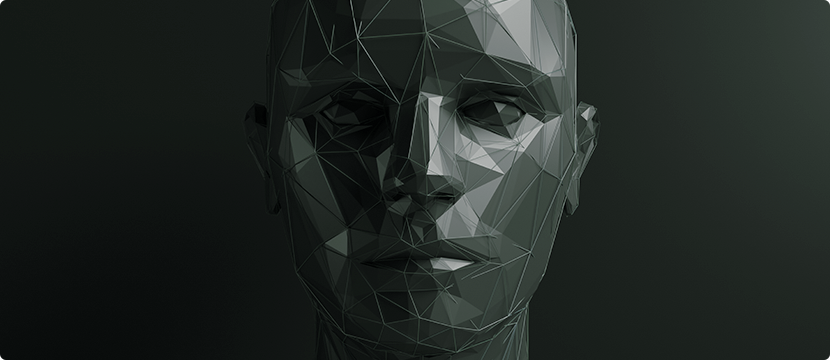
How is AI aiding hackers to commit crimes?
AI-based cyberattacks are on the rise by hackers. Hackers are upskilling themselves as technological advancement takes place by using AI which is faster, adaptable, and more efficient at attacking processes than traditional methods used by hackers. Let’s delve into various ML and DL-based algorithms and methodologies used by hackers to commit crimes.
-
Machine Learning for Web Application Security Exploits:
ML was first used in exploiting web application vulnerabilities like password brute-force attacks. A password brute-force attack is one in which a hacker can try trillions of passwords in less time against a system in an attempt to gain access. Hackers even started using botnets to increase their chances of success in brute force attacks. CAPTCHAs(Completely Automated Public Turing test to tell Computers and Humans Apart) are often used as a security control to avoid brute force attacks over the internet.
In Jan 2019, F-secure LABSⅳ team successfully cracked simple text-based CAPTCHA using their AI-based CAPTCHA cracking server called CAPTCHA-22. It uses ML modules like OpenCV, Computer Vision, and Attention-based OCR (AOCR) model, which uses a sliding convolutional neural network (CNN), and python frameworks. We can train deep convolutional neural net models to find the letters and digits in the CAPTCHA image.
In May 2020, the same F-secure Labsⅴ team was successful in bypassing CAPTCHA Outlook Web App (OWA) portal, where the noise level in the CAPTCHA was significant.
-
Machine Learning for DDOS Exploits:
A recent DDOS (Distributed Denial of Service) attack using AI-Controlled Botnet on TaskRabbit serversⅵ in April 2018 revealed 3.75 million users’ sensitive data like Social Security numbers and bank account details were scooped from their user data and before they could restore the website, an additional 141 million users were affected. Hackers are using ML to build out large-scale attack infrastructures, often referred to as bots or botnets, reflecting the automated nature of the attacks.
-
Machine Learning for Ransomware:
Cybersecurity firm Cybersecurity Venturesⅶ has predicted that, globally, businesses in 2021 will fall victim to a ransomware attack every 11 seconds, down from every 14 seconds in 2019. That figure is based on historical cybercrime figures. It is estimated that the cost of ransomware to businesses will top $20 billion in 2021 and that global damages related to cybercrime will reach $6 trillion. These ransomware attacks can increase multifold if at all hackers start planting AI-powered ransomware attacks.
IBMⅷ Research developed a new generation of malware called Deep Locker, which was a POC to raise awareness about AI-powered ransomware attacks. This Deep Locker can stealth under the radar and go undetected till its target is reached. It uses an Artificial Intelligence model to identify its target using indicators like facial recognition, geolocation, and voice recognition. The Trigger condition to unlock the attack is almost impossible to reverse engineer. The malicious payload will only be unlocked if the intended target is reached using a deep neural network (DNN) AI model.
-
Machine Learning for Social Engineering:
Social Engineering is the art of manipulating human psychology, which tricks users into performing actions in favor of hackers and gives away sensitive information to hackers.
In March 2019, an unusual cybercrimeⅸ case occurred in a U.K.-based energy firm where fraudsters used AI to Mimic CEO’s Voice. Criminals used AI-based software to impersonate a chief executive’s voice and demand a fraudulent transfer of €220,000 ($243,000).
Scams using Artificial Intelligence are a new challenge for companies to deal with. Hackers are also using AI to create deep fake videos of popular political icons and spreading them across social media to mislead people. Although it is not new to manipulate videos, AI technology is becoming very convenient for malicious intent personal to create deep fake videos using Deep-Neural Network based face swapping algorithms.
Loved what you read?
Get practical thought leadership articles on AI and Automation delivered to your inbox
Loved what you read?
Get practical thought leadership articles on AI and Automation delivered to your inbox
Advantages of AI in Cybersecurity
The power of Artificial Intelligence is “so incredible, it will change society in some very deep ways,” said billionaire Microsoft co-founder Bill Gates. AI-based security tools are much better than traditional security tools in finding anomalies & irregularities in network traffic or big data analysis, predicting malicious intent user behavior, identifying botnet programs, detecting malware, etc. They also can help organizations in timely detection and alerting of threats. So, all the use cases described in how hackers use AI to commit crimes can be prevented and remediated using AI technology itself. Deep fakes can be detected and prevented using Deep Learning techniques. It’s very important for organizations to embrace AI technology, understand how ML algorithms works and how they can enhance the security posture of an organization.
How to build 2X resilient, secure AI Systems?
Though there are many trending technologies like Bigdata, RPA, Cloud, 5G, IoT, and quantum computing, I see AI has emerged as a disruptive technology in this digital world. AI is used in every technology to solve worldly problems. A recent 2020 report from Statistaⅹ reveals that the global AI software market is expected to grow approximately 54% year-on-year and is expected to reach a forecast size of $22.6 billion.
Before an organization embraces any new technology, one must think through and consider all aspects cited below.
-
Are we using technology for ethical purposes to solve worldly problems?
-
What security measures will help to provide Confidentiality, Integrity, and Availability aspects?
-
What about privacy-related regulations and measures & legal laws?
I consider below cited security measures that can be leveraged while building applications/systems using AI.
-
Bring the resources up to speed by providing technical Training and Awareness
-
Perform Threat Modeling
-
Perform secure code review of software
-
Training the machine learning models on adversarial examples
-
Implement strong security controls like authentication and authorization along with other standard security controls
-
Implement Audit logging
-
Use of secure open-source components
-
Secure storage and transfer of data
-
Periodic checks — AI is not once set and forgotten
-
Most importantly, use AI-enabled solutions/tools to detect bot programs, threats & malicious activities, user behavioral analysis/network traffic analysis, and malware for timely detection and alerting
-
Perform pentesting of applications/systems
Conclusion
Enterprises should constantly study the evolution of AI technology, its capabilities, and techniques in order to identify and predict new threats and stay ahead of cybercriminals. Keep a tab on ever-evolving AI security standardsⅺ while embracing AI.
References:
- https://internetofbusiness.com/cambridge-analytica-vs-facebook-why-ai-laws-are-inadequate/
- https://www.cnbc.com/2019/04/03/chinese-hackers-tricked-teslas-autopilot-into-switching-lanes.html
- https://www.vox.com/future-perfect/2019/4/8/18297410/ai-tesla-self-driving-cars-adversarial-machine-learning
- https://labs.f-secure.com/blog/captcha22/
- https://labs.f-secure.com/blog/releasing-the-captcha-cracken/
- https://analyticsindiamag.com/5-artificial-intelligence-based-attacks-that-shocked-the-world-in-2018/
- https://www.natlawreview.com/article/ransomware-attacks-predicted-to-occur-every-11-seconds-2021-cost-20-billion
- https://securityintelligence.com/deeplocker-how-ai-can-power-a-stealthy-new-breed-of-malware/
- https://www.wsj.com/articles/fraudsters-use-ai-to-mimic-ceos-voice-in-unusual-cybercrime-case-11567157402
- https://www.statista.com/statistics/607716/worldwide-artificial-intelligence-market-revenues/
- https://cset.georgetown.edu/wp-content/uploads/t0121_AI_security_standardization_white_paper_EN.pdf